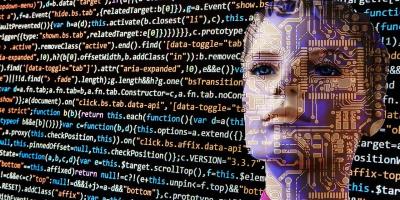
These days, ‘Technological Singularity’ is a buzzword among artificial intelligence (AI)-researchers and enthusiasts – a term used to identify that moment when AI will surpass human intelligence and force humans to bow before their artificial overlords.
The zeal to replicate the human in a machine is however based on a poor understanding about human attributes such as intelligence, consciousness, emotions, language etc., as very rarely do we come across AI-researchers who are invested in answering the fundamental questions about human capacities.>
In the absence of a much-needed groundwork, the comparison between human and artificial intelligence is based on a false assumption that the two are qualitatively the same. The dystopic moment of Technological Singularity in reality, is not round the corner.
AI-chatbot is a case in point. In recent years, it has contributed much to propagating the false narrative. These Large Language Models (LLMs) feed on enormous volumes of data, predominantly text, searching for and learning the most frequently attested patterns. Their seemingly language-like outputs are the creations of probabilistic techniques that predict the next word in a sequence based on its context.>
Continuous training with ever-expanding databases fine-tunes the models, progressively improving their ability to generate sentences that are accurate and contextually fitting. Not surprisingly, these machines are often flaunted as having not just achieved human-level skills including linguistic ones, but also outshining humans in artistic and poetic expressions, literature, scientific thought, and logical reasoning.
While such suggestions make fantastic topics for science fictions, the reality is very different. The linguist Noam Chomsky characterises AI-chatbots as high-end plagiarism machines. This character is the result of the probabilistic, frequency-based learning mechanisms that restrict the outputs of these machines to their databases, with their inputs exclusively determining their outputs.>
Aided with high-speed memories and processing powers, chatbots are master mimickers as they quickly learn and repeat the available patterns in the datasets; there is no originality and creativity in their behaviour.>
Human language, on the other hand, is creative, allowing us to form new structures that we have never heard or uttered before, and letting us imagine and talk about things and events beyond the immediate. Human language production is not restricted to what we hear or learn; we also create novel sentences, using a finite number of combinatorial rules of languages.
>Another major difference between the two comes in the manner of chatbots’ inability to distinguish possible linguistic strings from impossible ones. This distinction is central to human languages. Just as a human eye has a limited visual spectrum that prevents us from perceiving the world beyond certain wavelengths, human languages too have a limited number of possible combinations.
English, for instance, does not allow slp combinations, but has words with spl combinations (e.g., split). Some rules are also universal, as for instance no language attests equivalents of the bad English sentence, Who do you know the fact that John likes.>
Human languages are constrained, and human speakers naturally segregate the acceptable sentences from the unacceptable ones, even when these structures are not part of their received environmental input.>
Chatbots are incapable of such intuitions; if they are fed with larger sets of concocted unacceptable structures, they will end up generating unacceptable structures.>
Even kindergarten kids far exceed chatbots in linguistic competence. Language Acquisition research has established that human children do not rely on large datasets and token frequencies to acquire languages(s). In most cases, the data that young children are exposed to, are impoverished and incomplete (adult speech is often fragmented, and words are interspersed with pauses and interjections).
Yet, children can judge acceptable structures in their own language(s) much before they are introduced to grammar lessons in schools. Even the so-called errors found in child speech deviate from adult speech only as much as grammatical rules allow; child languages never exhibit impossible strings.>
Human language and intelligence are two tightly connected cognitive abilities. The ability for complex thoughts in humans and the remarkably quick progress in agriculture, science, technology, and art are often traced to the evolution of language in Homo Sapiens.>
Animals and birds do not have the same linguistic abilities and intelligence as humans, and no one honestly conceives of a day when our living ape relatives and neighbourhood birds will evolve human cognitive abilities. What, then, explains the irrational enthusiasm of AI-enthusiasts, and their conviction that AI has human-intelligence and will surpass humans in the future?
One reason for this rather unrealistic comparison is historical and can be traced back to the Turing Test proposed by Alan Turing (often known as the father of modern Computer Science) in his 1950 paper in the philosophy journal Mind. The Turing Test is a conversation between a human interrogator and another entity who is hidden from the direct sight of the interrogator and can be either a human or a machine.>
If the human interrogator fails to distinguish the responses of the two, at least 30% of the time, then it can be confirmed that there is no difference between humans and machines.>
Turing himself, though, never seriously considered this possibility, as is evident from his exchange with his friend Robin Gandy where he confides that he proposed the test more as a light-hearted propaganda, than as a topic for serious scientific investigation. Future researchers however accepted this as an ideal way to test intelligence based simply on the outputs of machines.
In their enthusiasm, they even overlooked the many limitations of the test. For one, the 30% threshold proposed by Turing is a mystery. There is also no suggestion on controlling the subjectivity involved in the evaluation by the human interrogator. There are no indications on the kinds of questions that should be asked, or on what count as intelligent answers.>
The other, and more important reason for why the narrative continues, is a confusion regarding the aims of AI. Margaret Boden, a prominent Cognitive Scientist and AI-researcher identifies two primary aims of AI. The first goal – the technological – is to use computers to do useful things and the second goal – the scientific – is to use AI concepts and models to understand humans and the world better.>
Technological advances in AI have been phenomenal in recent decades – today, it is present everywhere, including the home, the office, the hospital, the airport, the sky, the space, Mars etc.>
However, the scientific aim remains largely ignored, and questions of the underlying architectures and models are mostly pushed to the periphery. The fascination with AI outputs is enough to kill the excitement of enquiry regarding the computational mechanisms that generate different types of intelligent behaviour. This is a fine example of where the physical (i.e., the observable behaviour) trumps the abstract (i.e., the computations).>
One way to ameliorate this problem is to encourage interdisciplinary research in AI, by seriously considering the results of decades-long enquiries in psychology, linguistics, anthropology, philosophy, and neuroscience. These disciplines together constitute what is known as Cognitive Science, an enterprise that developed in the 1950s’ post-WWII era.>
Cognitive Science countered the then-prominent behaviourist approach to mind and cognition, by drawing upon the results from multiple domains of knowledge – behaviour, language, brain, computers, culture – to give holistic answers about humans and the world.>
To move away from the technological hype, AI must closely align itself with Cognitive Science. Only then, it will stop making incorrect observations about humans based solely on the surface behaviour of machines.>
Marvin Minsky, one of the pioneers of AI, wrote in a 1960 paper titled Steps towards Artificial Intelligence, “Should we ask what intelligence “really is”? My own view is that this is more of an aesthetic question, or one of sense of dignity, than a technical matter!”>
It is evident from this quote that the field lacked a clear understanding of the term ‘intelligence’ even back then, forcing Minsky to turn to aesthetics to defend the word ‘intelligence’ in ‘Artificial Intelligence’.>
Six decades on, research has made great advancements and we have a much better understanding of intelligence, learning and behaviour. We can use this knowledge to answer if machines are human-like intelligent, and if they can end up being more intelligent and powerful in the future. Till then, ‘Technological Singularity’ is a phrase that will continue to hoodwink AI-researchers themselves into not acknowledging the real challenges in the science of AI.>
Pritha Chandra is Professor of Theoretical Linguistics and Cognitive Science at the Indian Institute of Technology Delhi. She researches languages with the intent to understand how the human mind works.>