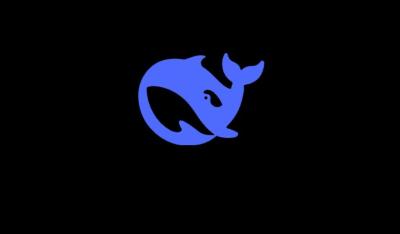
The knee-jerk reaction to the release of Chinese company DeepSeek’s AI chatbot mistakenly assumes it gives China an enduring lead in artificial intelligence development and misses key ways it could drive demand for AI hardware.>
The DeepSeek model was unveiled at the end of January, offering an AI chatbot competitive with the US’s OpenAI’s leading model, o1, which drives ChatGPT today.>
DeepSeek’s model offered major advances in the way it uses hardware, including using far fewer and less powerful chips than other models, and in its learning efficiency, making it much cheaper to create.>
The announcement dominated the international media cycle and commentators frequently suggested that the arrival of DeepSeek would dramatically cut demand for AI chips.>
The Deep Seek announcement also triggered a plunge in US tech stocks that wiped nearly AU$1 trillion off the value of leading chipmaker Nvidia.>
This dramatic reaction misses four ways DeepSeek’s innovation could actually expand demand for AI hardware:>
- By cutting the resources needed to train a model, more companies will be able to train models for their own needs and avoid paying a premium for access to the big tech models.
- The big tech companies could combine the more efficient training with larger resources to further improve performance.
- Researchers will be able to expand the number of experiments they do without needing more resources.
- OpenAI and other leading model providers could expand their range of models, switching from one generic model — essentially a jack-of-all-trades like we have now — to a variety of more specialised models, for example one optimised for scientists versus another made for writers.
What makes DeepSeek’s model so special?>
Researchers around the world have been exploring ways to improve the performance of AI models.>
Innovations in the core ideas are widely published, allowing researchers to build on each other’s work.
DeepSeek has brought together and extended a range of ideas, with the key advances in hardware and the way learning works.>
DeepSeek uses the hardware more efficiently. When training these large models, so many computers are involved that communication between them can become a bottleneck. Computers sit idle, wasting time while waiting for communication. DeepSeek developed new ways to do calculations and communication at the same time, avoiding downtime.
It has also brought innovation to how learning works. All large language models today have three phases of learning.>
First, the language model learns from vast amounts of text, attempting to predict the next word and getting updated if it makes a mistake. It then learns from a much smaller set of specific examples that enables the large language model to be able to communicate with users conversationally. Finally, the language model learns by generating output, being judged, and adjusting in response.
In the last phase, there is no single correct answer in each step of learning. Instead, the model is learning that one output is better or worse than another.>
DeepSeek’s method compares a large set of outputs in the last phase of learning, which is effective enough to allow the second and third stages to be much shorter and achieve the same results.>
Combined, these improvements dramatically improve efficiency.>
How will DeepSeek’s model drive further AI development?>
One option is to train and run any existing AI model using DeepSeek’s efficiency gains to reduce the costs and environmental impacts of the model while still being able to achieve the same results.>
We could also use DeepSeek innovations to train better models. That could mean scaling these techniques up to more hardware and longer training, or it could mean making a variety of models, each suited for a specific task or user type.>
There is still a lot we don’t know.>
DeepSeek’s work is more open source than OpenAI because it has released its models, yet it’s not truly open source like the non-profit Allen Institute for AI’s OLMo models that are used in their Playground chatbot.>
Critically, we know very little about the data used in training. Microsoft and OpenAI are investigating claims some of their data may have been used to make DeepSeek’s model. We also don’t know who has access to the data that users provide to their website and app.>
There are also elements of censorship in the DeepSeek model. For example, it will refuse to discuss free speech in China. The good news is that DeepSeek has published descriptions of its methods so researchers and developers can use the ideas to create new models, with no risk of DeepSeek’s biases transferring.>
The DeepSeek development is another significant step along AI’s overall trajectory but it is not a fundamental step-change like the switch to machine learning in the 1990s or the rise of neural networks in the 2010s.>
It is unlikely that this will lead to an enduring lead for DeepSeek in AI development.>
DeepSeek’s success shows that AI innovation can happen anywhere with a team that is technically sharp and fairly well-funded. Researchers around the world will continue to compete, with the lead moving back and forth between companies.>
For consumers, DeepSeek could also be a step towards greater control of your own data and more personalised models.>
Recently, Nvidia announced DIGITS, a desktop computer with enough computing power to run large language models.>
If the computing power on your desk grows and the scale of models shrinks, users might be able to run a high-performing large language model themselves, eliminating the need for data to even leave the home or office.>
And that’s likely to lead to more use of AI, not less.>
Dr Jonathan K. Kummerfeld is a senior lecturer in the School of Computer Science at The University of Sydney. He works on natural language processing, with a particular focus on systems for collaboration between people and AI models.>
Originally published under Creative Commons by 360info™.>